Plenary Lectures
Stefka Fidanova
Institute of Information and Communication Technologies
Bulgarian Academy of Sciences
E-mail: stefka@parallel.bas.bg
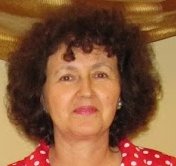
Ant Colony Optimization and Applications
Abstract. Collective intelligence allows animals to overcome difficulties and obstacles in nature, even when they do not have a high level of individual intelligence. Bees, ant colonies, bird , fish passages, etc. can be given as examples of group intelligence. This phenomenon has inspired scientists to imitate nature in their quest to solve complex problems coming from real life and industry. The unique behavior of ants in nature and their ability to always find the shortest path between the nest and the food source, gives the idea for the creation of the ants method. The application of ant colony optimization include knapsack problem, grid scheduling problem, GPS surveying problem, bioreactor modeling problem, wireless sensor network positioning, image edges detection, workforce planning, agricultural modelling and many others. The goal of this presentation is to show the diverse capabilities and applications of ant colony optimization.
Brief Biography of the Speaker: Stefka Fidanova is Professor of Computer Science at Institute of Information and Communication Technologies, Bulgarian Academy of Sciences. Her research interests include theory, methods, applications of combinatorial optimization and parallel algorithms. She heads the research group of Parallel Algorithms and Machine Learning. She has authored over 200 refereed journal, proceedings and collection papers, edited 13 proceedings, collections and special issues and written 2 monographs. She belongs to the editorial boards of several international journals. She has received the Career Award 2018 of Marie Curie Alumni Association of EU. She is listed in the World's Top 2% Scientists by Stanford University in 2021.
Magda Gregorová
Technical University of Applied Science Würzburg-Schweinfurt, Germany
E-mail: magda.gregorova@thws.de
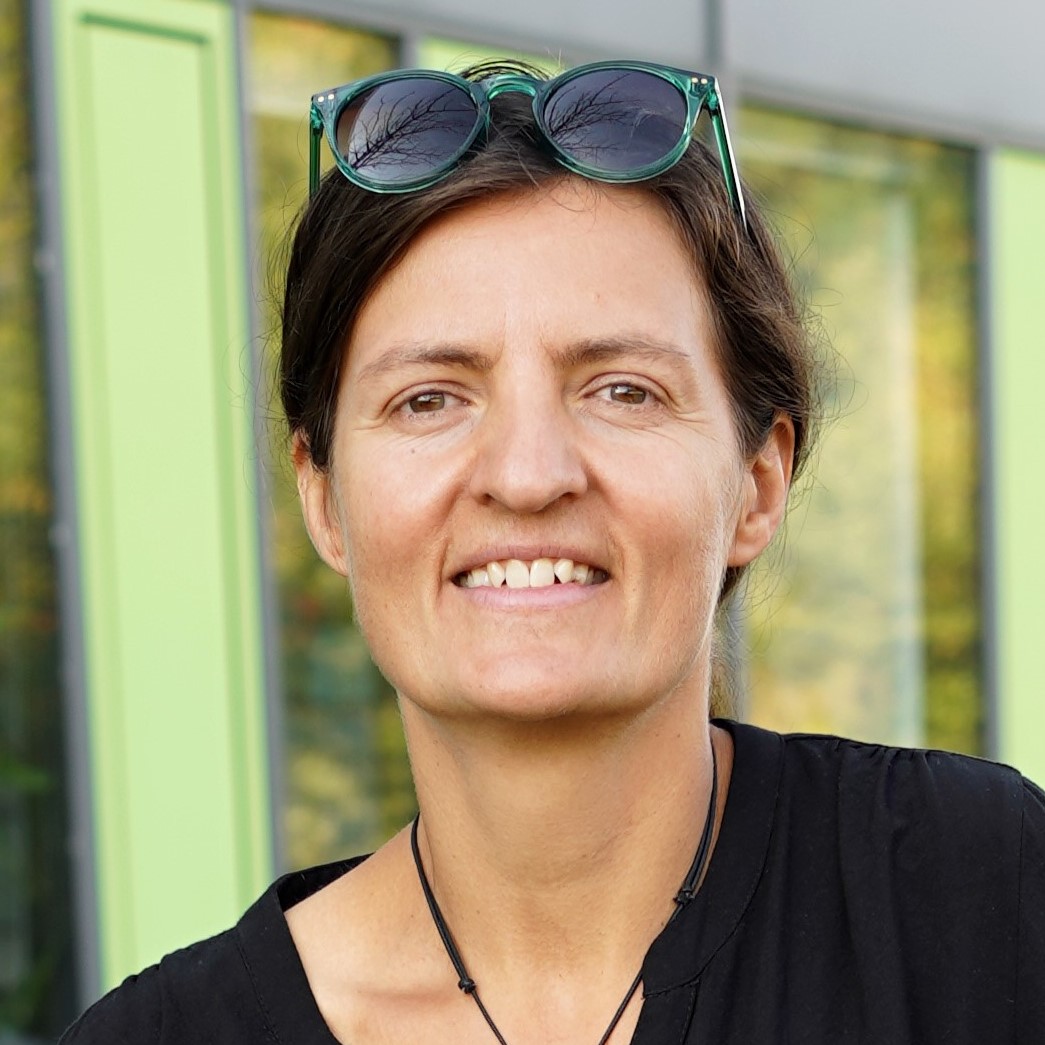
Deep generative modelling - beyond language and videos
Abstract. In this talk we will discuss deep generative models for other domains and purposes than generating seemingly realistic pieces of text, images or videos. We will explore a range of problems, where we use deep unsupervised methods to learn high-dimensional data distributions for various downstream tasks. In particular, we will investigate the problem of modelling complex relational structures represented as graphs and the challenges these pose for generating new graph examples (e.g. for de novo drug design), we will see how generative models can be used for explaining decisions of independent predictor models, and we will look at some examples of industrial applications such as deep generative modelling for optimization of electrical engine designs. We will conclude with some more general thoughts about the current state of research in deep generative modelling.
Brief Biography of the Speaker: Magda Gregorova comes from Prague, Czech Republic, where she obtained her Master‘s degree in Statistics (2001) from the University of Economics. She started her career of an applied statistician in the Czech National Bank, where she headed a technical unit on financial statistics and collaborated closely with the ECB and the IMF. After several years in banking she has decided to follow an international career and joined Eurocontrol, the European Organization for the Safety of Air Navigation based in Brussels, Belgium, as a statistical analyst and forecaster. She then moved to Geneva, Switzerland, where she obtained in 2018 a PhD in machine learning from the Computer Science Department of the University of Geneva. She continued as a post-doc in the Data Mining and Machine Learning group of the University of Applied Sciences of Western Switzerland. In 2021 she got the professorship for representation and learning at the Technical University of Applied Science Würzburg-Schweinfurt, Germany. Since 2022 she heads the research Center for Artificial Intelligence and Robotics (CAIRO) of the University. Her research focuses on deep generative methods for modeling complex high-dimensional distributions, in particular for discrete structures, and for learning useful representations for downstream tasks.
Andrei Paun
Faculty of Mathematics and Computer Science
University of Bucharest, Romania
E-mail: apaun@fmi.unibuc.ro
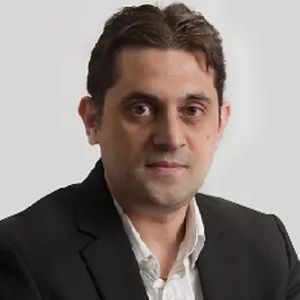
Abstract.
Brief Biography of the Speaker: Andrei received his BSc in Mathematics with a major in Computer Science from University of Bucharest (Romania) and later he received a Master’s followed by a PhD in Computer Science from University of Western Ontario (Canada) in 2003. His PhD supervisor was Dr. Sheng Yu, from whom he “inherited” the interest in Finite Automata and OOP modeling as well as the Biocomputing research field that was the main thrust of his PhD dissertation. Following the PhD Andrei was a postdoc at Technical University of Wien (Austria) and later at University of Rovira i Virgili (Spain). He then moved to the US as an assistant and later associate professor of Computer Science (tenured) at Louisiana Tech University (USA). He later returned to Europe having positions at University of Western Ontario (Spain), University of Bucharest (Romania) and National Institute of R&D for Biological Sciences (Romania).
His main interests are Biocomputing and Bioinformatics as well as finite automata and other related areas.
Milan Tuba
Singidunum University
Belgrade, Serbia
E-mail: tuba@ieee.org
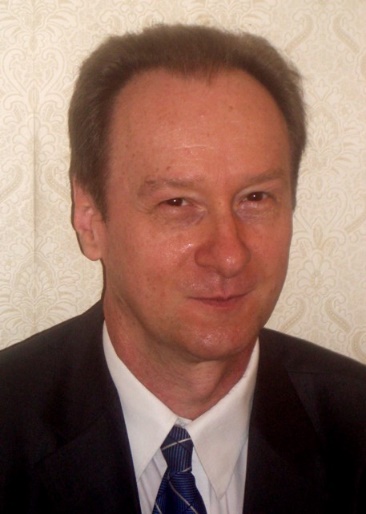
Abstract.
Brief Biography of the Speaker:
Milan Tuba is the Vice Rector for International Relations, Singidunum University, Belgrade, Serbia and was the Head of the Department for Mathematical Sciences at State University of Novi Pazar and the Dean of the Graduate School of Computer Science at John Naisbitt University. He is listed in the World's Top 2% Scientists by Stanford University in 2020 and 2021. Prof. Tuba is the author or coauthor of more than 250 scientific papers (cited more than 5000 times, h-index 42) and editor, coeditor or member of the editorial board or scientific committee of number of scientific journals and conferences. He was invited and delivered around 60 keynote lectures at international conferences.
He received B. S. in Mathematics, M. S. in Mathematics, M. S. in Computer Science, M. Ph. in Computer Science, Ph. D. in Computer Science from University of Belgrade and New York University. From 1983 to 1994 he was in the U.S.A. first at Vanderbilt University in Nashville and Courant Institute of Mathematical Sciences, New York University and later as Assistant Professor of Electrical Engineering at Cooper Union School of Engineering, New York. During that time he was the founder and director of Microprocessor Lab and VLSI Lab, leader of the NSF scientific projects and theses supervisor. From 1994 he was Assistant Professor of Computer Science and Director of Computer Center at University of Belgrade, from 2001 Associate Professor, Faculty of Mathematics, University of Belgrade, from 2004 also a Professor of Computer Science and Dean of the College of Computer Science, Megatrend University Belgrade. Prof. Tuba was the principal creator of the new curricula and programs at the Faculty of Mathematics and Computer Science at the University of Belgrade and later at John Naisbitt University where he was the founder and practically alone established a complete new school with bachelor, master and PhD program. He was teaching more than 20 graduate and undergraduate courses, from VLSI Design and Computer Architecture to Computer Networks, Operating Systems, Artificial Intelligence, Image Processing, Calculus and Queuing Theory.
His research interest includes nature-inspired optimizations applied to image processing, computer networks, and neural networks. Member of the ACM, IEEE, AMS, SIAM, IFNA, IASEI.